Focus on problems in the smart electric grid where much of the data infrastructure is in place and evaluation of developed methodologies
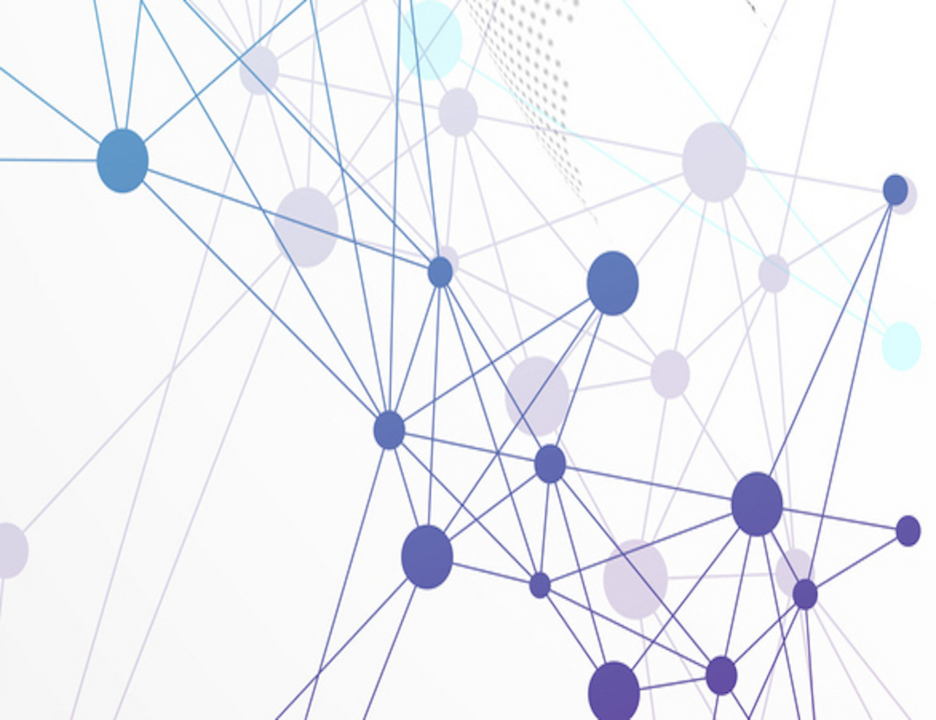
As infrastructural systems rapidly grow in scale, complexity and interconnectedness, legacy operations driving the systems have shown to be limited in their ability to handle large-scale disasters that can occur either through natural extreme events, or through carefully orchestrated attacks, and in particular cyber-attacks. Advances in sensing and communication technologies allow diverse data collection at massive scale, granting unprecedented visibility into system operations and provide enormous potential to overhaul the operational paradigms towards increased safety, security and sustainability. It is towards this end that we are conducting data-intensive data-driven research for inference and control in large scale cyber physical infrastructural networks. Our research builds on four broad thrusts:
- Machine learning for cyber physical resilience.
- Physics-informed graph learning and inference.
- Explainable graphical model inference.
- Reinforcement learning for distributed control
- Unified data representation framework through knowledge graphs.
Research Focus Group Members
This is a new initiative and collaborative research group. The pilot phase will focus on robust and explainable physics-informed graph neural networks for smart transportation systems under extreme conditions such as natural disasters.
- Rick Blum, Computer Science & Engineering
- Paolo Bochinni, Civil & Environmental Engineering
- Brian Davison, Computer Science & Engineering
- Jeff Heflin, Computer Science & Engineering
- Jessecae Marsh, Psychology
- Ted Ralphs, Industrial & Systems Engineering
Parv Venkitasubramaniam, Electrical & Computer Engineering