Use of machine learning and deep learning. for modeling complex physical systems of materials and chemical processes
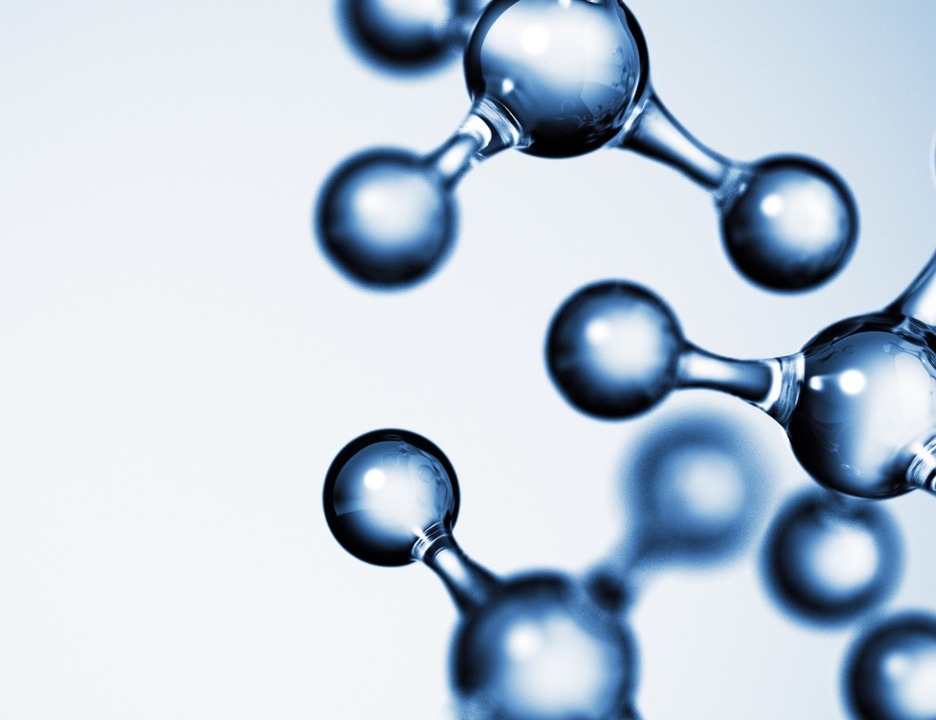
Research Focus Areas
Data science has become ubiquitous in science and engineering. There is burgeoning activity in the adoption of machine learning tools in physics, chemistry, chemical engineering, materials science, and related disciplines to elucidate and design complex processes (chemical/biological, engineered/natural) or material systems with wide ranging applications addressing grand challenges in energy, health, environment, and water. At Lehigh, data science and machine learning tools are being used for modeling of complex molecular and material systems of physical, chemical or biological origin to discover the underlying synthesis-structure-property relationships and leverage them in inverse design of new materials and molecules.
Research Group Members
-
Ganesh Balasubramanian, Mechanical Engineering & Mechanics, RCEAS
-
Chinedu Ekuma, Physics, CAS
-
Lisa Fredin, Chemistry, CAS
-
Anand Jagota, Chemical & Biomolecular Engineering / Bioengineering, RCEAS
-
Srinivas Rangarajan, Chemical & Biomolecular Engineering, RCEAS
-
Edmund Webb III, Mechanical Engineering & Mechanics, RCEAS