PhD student Amin Hosseiny Marani
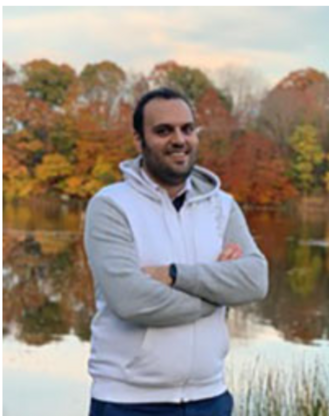
PhD student Amin Hosseiny Marani
One Rating to Rule Them All?: Evidence of Multidimensionality in Human Assessment of Topic Labeling Quality
PhD student Amin Hosseiny Marani recently co-authored a paper with his advisor, I-DISC faculty member Eric Baumer. The paper investigates how humans evaluate the quality of labels assigned to a topic (for example, in a news headline). Amin and his coauthors conclude that humans make such assessments in nuanced ways. Their findings have implications for natural language processing (NLP) and for machine learning (ML) systems more broadly.
Abstract: Two general approaches are common for evaluating automatically generated labels in topic modeling: direct human assessment; or performance metrics that can be calculated without, but still correlate with, human assessment. However, both approaches implicitly assume that the quality of a topic label is single-dimensional. In contrast, this paper provides evidence that human assessments about the quality of topic labels consist of multiple latent dimensions. This evidence comes from human assessments of four simple labeling techniques. For each label, study participants responded to several items asking them to assess each label according to a variety of different criteria. Exploratory factor analysis shows that these human assessments of labeling quality have a two-factor latent structure. Subsequent analysis demonstrates that this multi-item, two-factor assessment can reveal nuances that would be missed using either a single-item human assessment of perceived label quality or established performance metrics. The paper concludes by suggesting future directions for the development of human-centered approaches to evaluating NLP and ML systems more broadly.